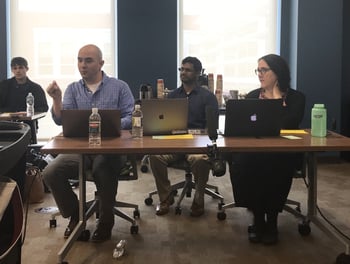
Two of our Data Science bootcamp students, Evan Lancaster and Mahesh Rao, and our Data Science instructor, Mary van Valkenburg, were invited to present at the inaugural Metro Data Day last Friday. Metro Data Day is a way for Metro Nashville to gather data champions from across all agencies of the city government to learn.
Keith Durbin, Metro Nashville’s CIO, opened the meeting by sharing about Nashville’s Smart City Projects and how data will help them make better decisions to serve the Nashville community.
Robyn Mace, Metro Nashville’s CDO (Chief Data Officer), then kicked off the Inaugural Metro Data Day. Last fall, Robyn spoke with our Data Science cohort about Metro open data and provided a data question. She wanted to reduce over 750 property violation categories to less than 20. She shared with students strategies to consolidate the categories and apply the new categories to the open dataset of property violations.
After an introduction from Mary about NSS and the Data Science bootcamp, Evan and Mahesh shared the insights they gained from their work with the Metro Nashville property violations data project.
Cleaning The Data
Evan started by walking the audience through the cleaning process for the list of code violations. The process included pulling only the necessary fields and dropping NULL values. He then explained how he approached simplifying the 750 categories down to less than 20. The advice he received was to pick a place and simply start. Once he assigned a category to a code, he filtered them out, slowly reducing his list.
Natural Language Processing
With categories in place, he now faced the challenge of categorizing each violation. He noticed that the violation data included descriptions that, most of the time, used words from the code that was in violation. Since he already had categories for the codes, he turned to Natural Language Processing (NLP) to identify and categorize codes. He used two-thirds of the property codes dataset to train NLP and tested it on the remaining third.
NLP was more accurate for some categories versus others. After reviewing the data, Evan realized that if he was going to make it more accurate, he would have to train it for the edge cases. With an accuracy of 75-80%, he decided that improving the accuracy for edge cases was not the best use of time. He explained how accurate you need to be depends on the type of data you’re using. For example, if you were using NLP for cancer research, you would want your accuracy levels to be very high.
NLP was then applied to the open dataset of property violations over a two year period.
Gleaning Insights Through Visualizations
Mahesh walked us through his visualizations of the data. One visualization showed the location of the property violations. When he reduced the violation categories shown to only “signs,” you could clearly see that most of these violations occur on the major roads in Nashville.
He also showed a strip plot marking each violation for handful of categories over a two year window. That data showed that lawn care violations are seasonal, with few incidents occurring during the winter months. It also showed a rise of violations from short-term rentals as they have grown in popularity. While these align with what one might expect, it’s important to have data to back up our expectations.
How Metro Nashville Can Use This Data
Our Data Science bootcamp has been fortunate to work with real-world data provided by organizations across Nashville. This data enriches their learning experience by working with realistic, messy datasets that they will encounter in their jobs. But using real-world data is not just a benefit for our students. It can benefit the organization that provided the data as well.
The agency representatives in attendance were excited about the results and how this data can be used in their jobs. They provided some examples of how this information help them.
- Provide updates to city council members on code violations in their districts
- Allocate resources to code inspections. For example, they may bring on additional help from May-September when there is a rise in lawn care violations or dedicate more resources to short-term rental violations.
- Help different agencies within the city work together and build efficiencies, such as the Fire Department working with the Department of Codes and Building Safety.
While the scope of the project within the class did not involve a working application with a user interface, our hope is that we can continue to work with Metro as they advocate for the use of data across the city and their agencies.